Our Mission
Our mission is to utilize eye-tracking scanpaths and machine learning technology to make ASD diagnoses more accessible in the U.S.
The Problem
Families embarking on an ASD diagnosis journey find themselves facing a 2 year process on average, losing valuable, impactful time for early intervention resources. Seeking a pediatric ASD diagnosis involves a variety of screening tests in several format, which can add up to anywhere from $1,000 to $2,000 in the U.S. Diagnoses can only be provided by specialized medical and psychiatric personnel, severely limiting appointment availability and extending wait times. Without an official diagnosis, many therapies and specialist referrals are inaccessible and unable to be covered by most insurance, further exacerbating the financial burden on families.​
Our Solution
By utilizing a public eye-tracking scanpath dataset and machine learning technology, we've deployed a Provider Portal prototype product to augment the ASD screening process. Our tool consists of a client user interface where a scanpath image is loaded by the provider. The image is then sent to our image classification API in Amazon EKS. The trained model processes the image, performs a prediction, and returns the findings alongside the explanation for the classification to the provider portal page. An intuitive, seamless, upload interface will allow medical professionals to interpret session results quickly to make decisions more efficiently.

Technical Approach Overview
With a dataset of approximately 530 eye-tracking scanpaths, our team explored various machine learning models to find the most effective approach to distinguishing between scanpaths of children with and without ASD.
​
​
​
​
​
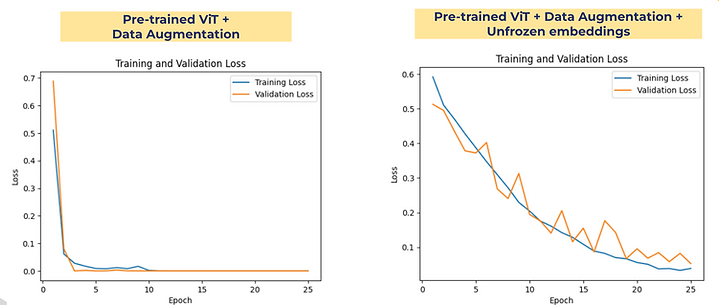
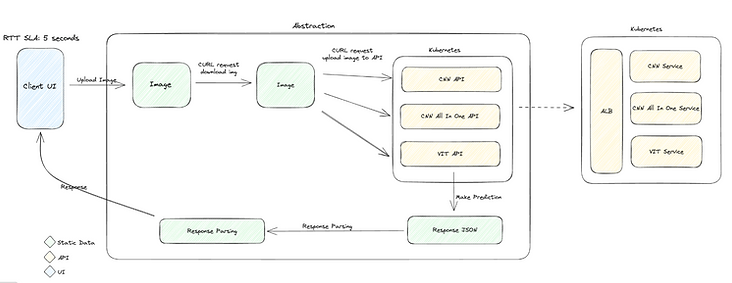
Potential Impact
Half of the children in the U.S. are currently being screened for ASD, with an estimate of 5 million children as risk for developmental delays. This population can be directly benefited by the burgeoning use of machine learning and AI alongside the professional screenings and interpretations of medical and psychiatric personnel. Research has shown that early intervention techniques, such as applied behavioral analysis sessions, are most impactful for children under the age of 5, further highlighting the importance of early diagnoses. By introducing eye-tracking machine learning classification alongside in-person diagnostic screenings, families can begin to receive diagnoses for their child well before the current 4-year-old average, resulting in more effective treatment, societal inclusion, and benefiting communities as a whole.
Team and Acknowledgements
Meet the team that built the Shine with Eyes prototype! We are a group of U.C. Berkeley MIDS graduate students in the Class of Fall 2023 who gathered to build Shine with Eyes as our final capstone project.
​
​
​
​
​
​
​
​
​
We would also like to thank our professors and fellow students at U.C. Berkeley, as well as the subject matter experts, psychiatrists, psychologists, and data scientists for their invaluable feedback, guidance, and insight​.
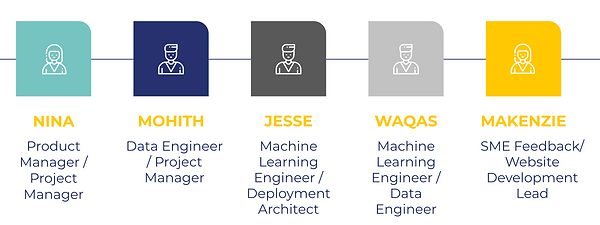